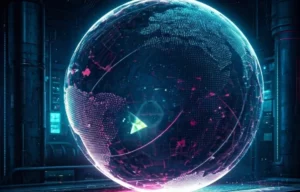
Prefer to listen instead? Here’s the podcast version of this article.
In the ever-expanding landscape of artificial intelligence, one emerging topic has been sparking significant debate: AI’s role in energy efficiency. On one hand, AI technologies promise to optimize energy use across industries, cut emissions, and enable smarter energy systems. On the other, concerns are mounting over the energy demands of training large AI models, which often require vast computational resources. As someone deeply engaged in exploring the intersection of AI and its practical impacts, I wanted to dive into this topic with you—let’s explore the potential, the challenges, and the path forward.
Â
AI is already transforming the energy sector by helping companies monitor consumption, reduce waste, and implement green technologies. For instance, AI algorithms are being used to predict energy demand patterns and adjust supply dynamically. This not only prevents energy overproduction but also ensures more efficient grid management.
Â
In renewable energy, AI plays a critical role in enhancing efficiency. For example, wind turbine optimization algorithms and solar panel maintenance powered by machine learning are revolutionizing the way renewable energy is harvested and managed. According to [Forbes], energy firms using AI are seeing operational efficiencies increase by up to 25%.
Â
Ironically, the very technology designed to save energy can also guzzle vast amounts of power. Training massive AI models—think of GPT-4 or Google’s Gemini—requires immense computational power, often generated by carbon-intensive energy sources. A report from MIT Technology Review revealed that the carbon footprint of training one large model could be comparable to the lifetime emissions of five cars.
Â
While companies like Google are investing in renewable-powered data centers, the reality remains that scaling AI sustainably will require a shift in how data centers operate globally. This is where initiatives like Nvidia’s energy-efficient GPUs, discussed in [Investopedia], become vital in reducing the energy footprint of AI computation.
Â
But let’s not lose hope—AI also presents us with ways to tackle its energy challenges. Machine learning algorithms can help data centers optimize cooling systems, which are a major energy drain. DeepMind, a subsidiary of Alphabet, famously cut its energy use for cooling by 40% using AI, as highlighted in this [FT article].
Â
Moreover, AI’s ability to optimize logistics and manufacturing can contribute to more sustainable production cycles, reducing overall emissions in supply chains. AI-driven insights into material sourcing, transportation routes, and factory energy management are now standard practices in cutting-edge industries.
Â
As AI’s energy consumption grows, regulators and industry leaders must collaborate to set ethical guidelines and invest in sustainable solutions. For instance, OpenAI’s development of more energy-efficient models, such as its o3 series highlighted by [Wired], signals a shift toward energy-conscious AI innovation.
Â
Governments are stepping in as well. The European Union’s Digital Decade initiative emphasizes green AI and sustainable data usage, which may set the global benchmark.
Â
AI’s role in energy efficiency is a tale of two narratives: one of immense promise and another of pressing responsibility. On the one hand, AI has the power to revolutionize energy systems, optimize resource use, and drive a greener future. On the other hand, the energy demands of building and deploying advanced AI systems challenge us to rethink how we innovate sustainably.
Â
The path forward calls for collaboration between tech innovators, policymakers, and industries to harness AI’s potential while minimizing its environmental impact. As we navigate this exciting but complex terrain, we must balance progress with responsibility, ensuring that AI remains a tool for empowerment, not a drain on our planet’s resources.
WEBINAR